Extensible Binary Encoding with CBOR
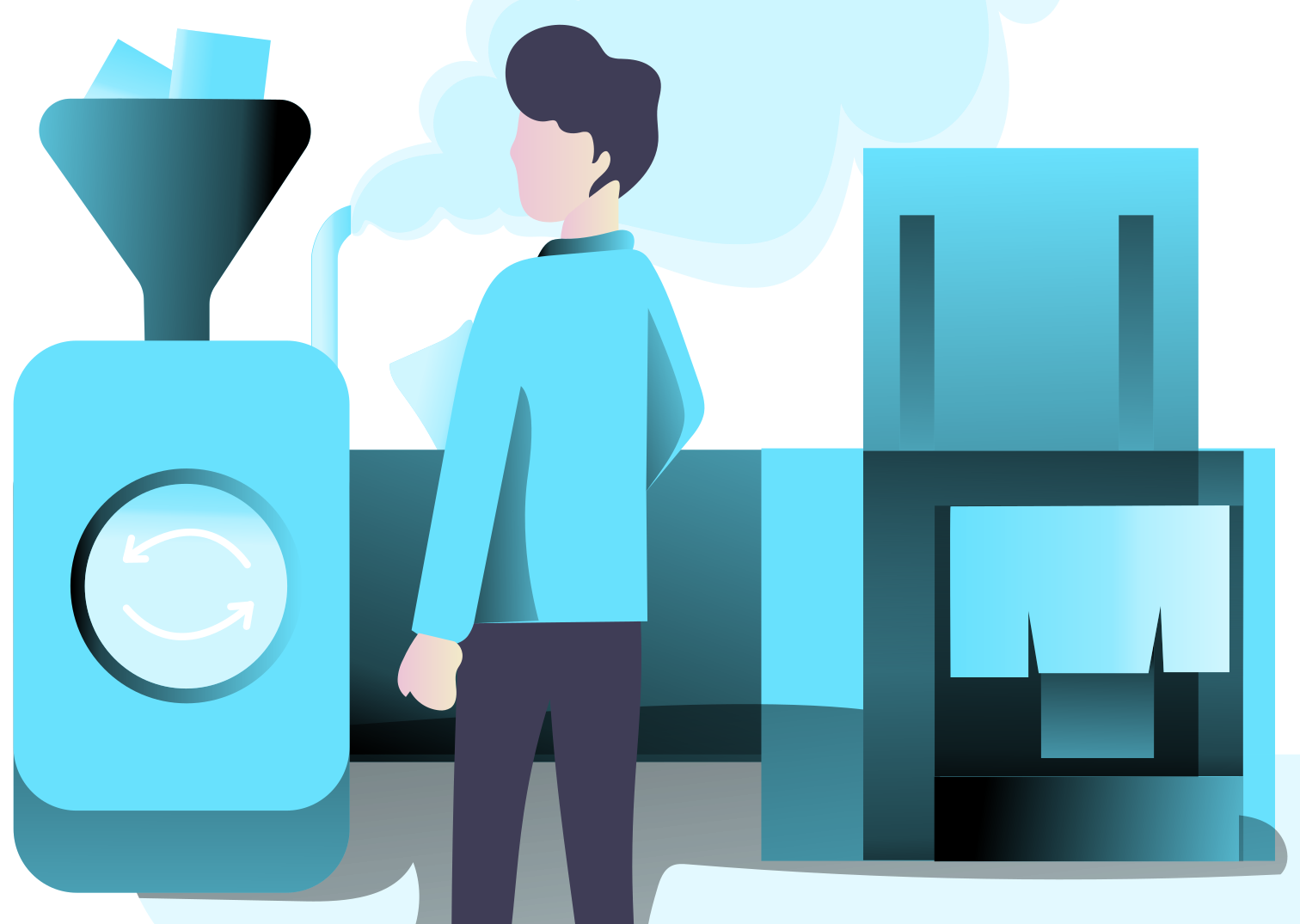
CBOR is a relatively new IETF draft standard extensible binary data format. Compared to similar formats like MessagePack and BSON, CBOR was developed from the ground up with clear goals:
- unambiguous encoding of most common data formats from Internet standards
- code compactness for encoder and decoder
- no schema description needed
- reasonably compact serialization
- applicability to constrained and unconstrained applications
- good JSON conversion
- extensibility
—RFC 7049 Appendix E, Copyright © 2013 IETF Trust, Bormann & Hoffman
In the context of data storage and messaging, most developers can relate to CBOR as a binary drop-in replacement for JSON. While CBOR doesn’t share the human readability of JSON, it can efficiently and unambiguously encode types of data that JSON struggles with. CBOR can also be extended with tags to optimize serialization beyond its standard primitives.
Encoding Binary Data
JSON is a ubiquitous data format for web and beyond, for many good reasons, but encoding blobs of binary data is an area where JSON falters. For example, if you are designing a JSON protocol to wrap the storage or transfer of arbitrary objects, your options are:
- Require that all input …
performance optimization browsers scalability nodejs benchmarks
The flow of hierarchical data extraction
1. Problem statement
There are many cases when people intend to collect data, for various purposes. One may want to compare prices or find out how musical fashion changes over time. There are a zillion potential uses of collected data.
The old-fashioned way to do this task is to hire a few dozen of people and explain them where should they go on the web, what should they collect, how they should write a report and how they should send it.
It is more effective to teach them this at the same time than to teach them separately, but even then, there will be misunderstandings, mistakes with high cost, not to mention the limit a human has when processing data in terms of the amount to process. As a result, the industry strives to make sure this is as automatic as possible.
This is why people write software to cope with this issue. The terms data-extractor, data-miner, data-crawler, data-spider mean software which extracts data from a source and stores it at the target. If data is mined from the web, then the more-specific web-extractor, web-miner, web-crawler, web-spider terms can be used.
In this article I will use the term “data-miner”.
This article deals with the extraction of …
data-mining machine-learning data-processing
Converting GraphQL Ruby Resolvers to the Class-based API
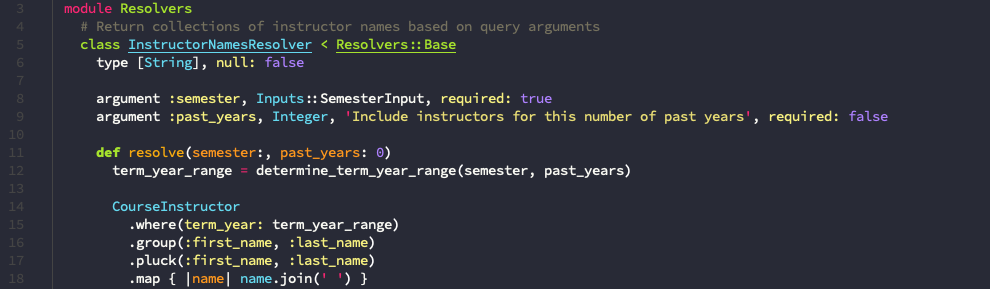
The GraphQL gem is a great tool for any Rails developer who wants to add a full-featured GraphQL API to their Rails applications.
I have been using GraphQL to serve an API in one of my Rails applications since late 2017 and have been very happy with the features and performance provided by the gem, but some of the domain-specific syntax for building out my API schema never felt quite right when compared to the other Ruby code I was writing in my projects. Fortunately, the 1.8.0 release of the GraphQL Ruby gem brought with it a new default class-based syntax while remaining compatible with existing code that predated the change.
The Class-based API guide that accompanied the changes does a good job of describing the upgrade path for developers who need to convert their existing schemas. The old .define
syntax is eventually going to be removed with version 2.0 of the gem, so I was interested in converting my existing API over to the new style, both to see what benefits the newer syntax provides and to ensure that the API schema remains compatible with future releases of the gem.
The GraphQL gem provides some rake tasks like graphql:upgrade:schema
and graphql:upgrade:member
for …
ruby graphql api
OWASP Top Ten Application Security Risks
I don’t consider myself a security expert. Still, to my surprise, I was asked to give a talk about security to all the End Point developers. Obviously I realized too late what I was getting myself into! Such an audience is not only pretty large, it is also challenging, many are more competent than me, and the risk to bore them is very high. Yet, the slides were prepared, the talk was given and the feedback was good.
It goes without saying that a broad, generic training about security, which still can give something to the listener, can’t be really improvised.
The platform for the talk was the OWASP Top Ten 2017 Project, which discusses the most critical security risks to web applications.
OWASP stands for Open Web Application Security Project and describes itself as “an open community dedicated to enabling organizations to develop, purchase, and maintain applications and APIs that can be trusted.” Its website provides plenty of resources to the developers.
The Top Ten consists of 10 broad classes of vulnerabilities. The data behind that comes from specialized firms and surveys, gathering information from 100,000 real-world applications and APIs. Some of these classes are very …
security
Volunteer While You Work From Home
I’ve always been an animal lover. I’ve currently got a dog, two cats, bees, and a flock of chickens in my tiny suburban home and I would get more if I could. Over the past few years I’ve taken up an interest in fostering animals through our local animal shelter. Above are our current fosters, two St. Bernard mix parvo pups.
I’m always looking for opportunities to do something in the community but as a busy mom with a full-time job, it can be difficult to fit volunteering into your schedule. What I’ve discovered is that animal fostering is a great volunteer job for someone that works from home.
How Does It Work?
Our local shelter has a list of foster volunteers who’ve completed their application process and requisite trainings. When they have an animal or group of animals that needs to be out of the shelter for a certain amount of time, they email everyone with a description of the foster. You review the information and decide if you’re a good fit.
We’re very lucky in that our local humane society provides all the food, bedding, medicine, and instruction you need. Your job is to take care of the animal or litter, report back to the shelter as needed, and return the animal when it …
remote-work community
Where are you with your Windows OS in 2019?
Photo by bradleypjohnson · CC BY 2.0
It should be of little surprise that on January 14, 2020, after a decade of Windows 7, Microsoft will stop providing security updates and support for this older operating system. Windows 7 was released in 2009, and due to its stability enjoyed many years as the go-to operating system for home and business alike.
Even now, it is estimated by NetMarketShare that over 40% of businesses still rely on it. Despite Microsoft having ended mainstream support for Windows 7 in 2015, it still offered extended support because of the operating system’s popularity, and the generally slow adoption of newer releases. However, that support shall soon end, as will support for Windows Server 2008 R2 (release 2), which also remains in wide use. Organizations of all kinds will need to upgrade to newer operating systems to remain secure.
The corporate adoption of Windows 8 and 8.1 may have been slow in part due to Microsoft’s radical changes to the user interface, such as replacing navigation menus with information-filled “live” tiles. Windows 10, however, was designed as a compromise, providing a Windows 7-like Start menu, while preserving the live tile interface …
windows security
Camping in the Clouds with Terraform and Ansible
Photo by Andrew E. Larsen · CC BY-ND 2.0
Right, so, show of hands: How many of you work on some bit of web code by doing a git clone
to your own laptop, developing the feature or bug fix, running through manual testing of the app until you’re happy with it, and off it goes back up to the repo when done? I’m curious, and I have a few questions for you:
- Have you ever had a bit of code that worked locally, but didn’t in production because of some difference in systems, dependencies, or something else in the stack?
- How do you show off your work to a client or management for approval? Can you demo several alternate changes to the same site at the same time?
- How do you bring in coworkers to “look over your shoulder“ and help with something, especially ones that are far away?
- How do you get a new coworker up to speed if they’re doing development themselves?
- If you’re working on multiple things, do you create multiple clones?
- How’re your backups?
Are you fidgeting nervously thinking about that? Sorry. ☹ But also, check out this little thing: DevCamps. It’s been an End Point staple for quite a while now, so if you’ve read our blog before you might have heard about it.
Long story short: In …
camps cloud development terraform ansible
Adding Awesomplete to Vue Components
IBM Model M SSK by njbair, used under CC BY-SA 2.0 / Cropped from original
Awesomplete is an “Ultra lightweight, customizable, simple autocomplete widget with zero dependencies, built with modern standards for modern browsers.”
Awesomplete caught my attention when I was looking for a lightweight autocomplete implementation to add to an existing, heavily styled form in a Vue.js single-file component. There are no fewer than 10 options on the Awesome Vue.js list of autocomplete libraries, but many of them brought their own dependencies or custom styling and I was looking for something simpler to add autocomplete features to my form.
I have created a live JSFiddle demo showing an implementation of Awesomplete in a Vue.js app, but the remainder of this post contains more details about adding Awesomplete to a single-file component in a larger Vue application.
Here is a screenshot and sample code for a simplified version of the Vue single-file component that I was working with:
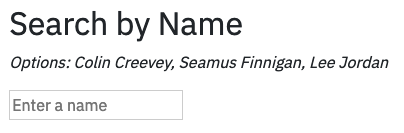
<template>
<div>
<h2>Search by Name</h2>
<p>
<em>
Options: {{ names.join(', ') }}
</em>
</p>
<form> …
vue javascript